The policy and governance landscape for artificial intelligence (AI) is multifaceted and complex due to several factors. First, a variety of actors at local, national, regional, and global levels contribute to the governance of AI. Second, the umbrella term “AI” itself spans diverse technologies, from robots to large language models (LLMs), necessitating a broad spectrum of regulations that impact AI across its lifecycle—design, development, and deployment.
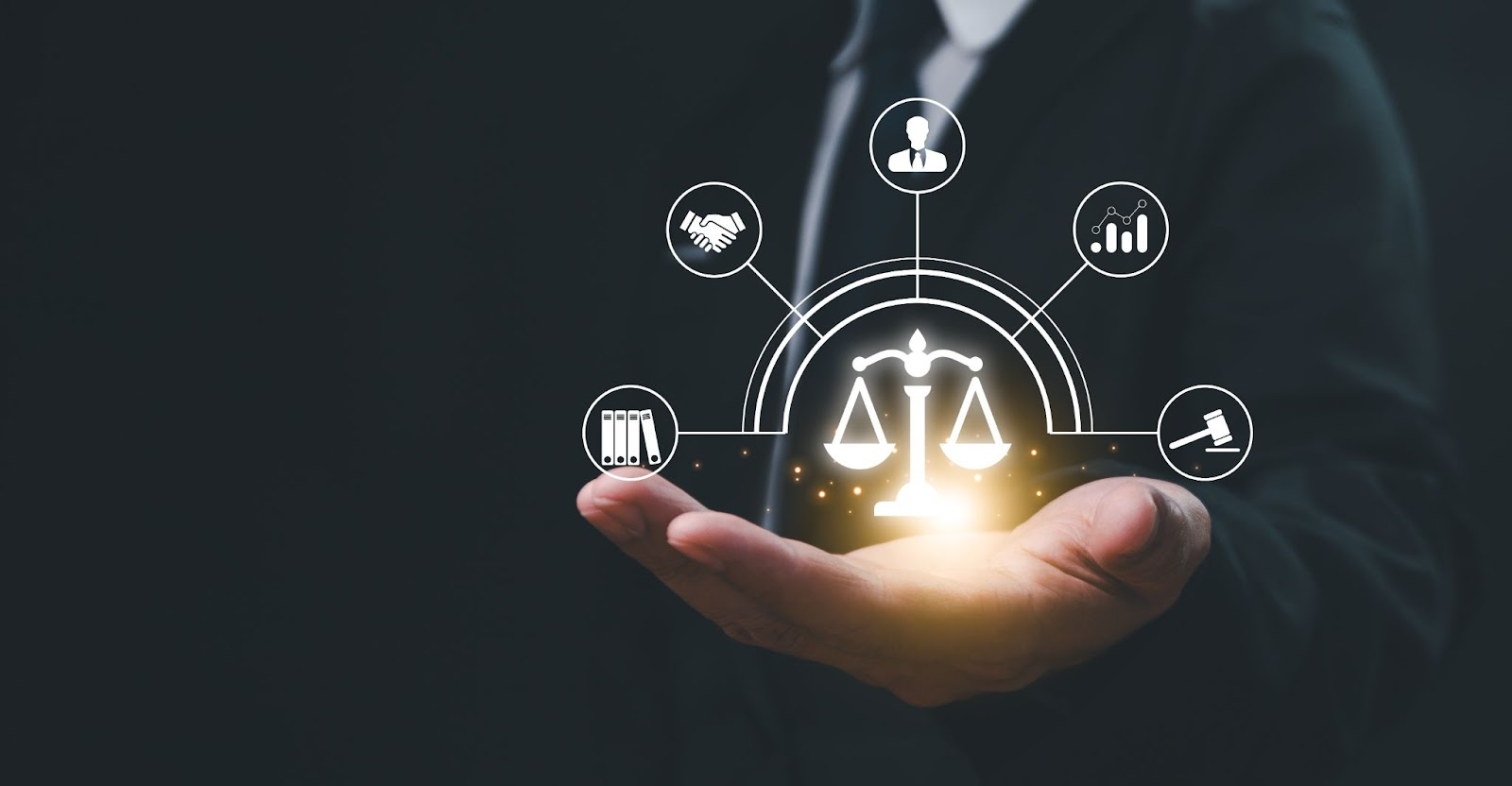
3.1 Understanding the Policy and Regulatory Pillar of AI
During the design and development phases, concerns about data provenance, bias, and transparency are predominant. The deployment phase focuses on preventing unethical uses, such as AI, in mass surveillance systems targeting political dissidents. This results in an intricate array of rules by various authorities governing AI's lifecycle, creating complexity but also enhancing predictability while ensuring safety and accountability.
To get a better understanding of the governance challenges posed by AI, AI can be categorized into foundation models, AI-powered physical products, small-scale AI as a service, and militarily relevant AI, each presenting unique risks and requiring specific governance strategies. Foundation models (large-scale AI models trained on vast amounts of diverse data, capable of performing a wide range of tasks)known for their scale and versatility, pose significant challenges, such as the potential misuse by malicious actors and complications arising from their evolution towards multi-modal capacities that inch towards artificial general intelligence. Safety summits and proactive regulations are crucial in addressing these concerns.
AI also permeates physical products, from everyday items like appliances to critical devices such as medical equipment, necessitating robust safety standards and cyber resilience. The distinction between high-risk and low-risk AI applications helps in tailoring governance appropriately. For digital AI services, which easily cross jurisdictions, international cooperation is essential to manage risks related to user safety and privacy.
In the military sphere, AI enhances capabilities from logistics to combat operations, raising ethical issues regarding human oversight and conflict escalation. The diverse applications of AI across these categories underscore the need for comprehensive and adaptable governance frameworks to mitigate risks while fostering innovation.
To address these diverse governance challenges, countries and regions have developed varying regulatory frameworks tailored to their specific priorities and concerns regarding AI development and deployment.
3.2 Current Regulatory Frameworks
While there are many private and multi-stakeholder initiatives formulating AI policy and governance frameworks, only governments and the European Commission have the power to make general laws and regulations that are automatically and directly binding on their populations. Below is an overview of the most important governmental initiatives in the realm of AI policy and governance.
To address these diverse governance challenges, countries and regions have developed varying regulatory frameworks tailored to their specific priorities and concerns regarding AI development and deployment.
3.2.1 Overview of Existing Frameworks
In August 2024, the European Union signed the AI Act, the world’s first comprehensive legal framework for AI. The AI Act uses a risk-based approach, meaning that AI applications are regulated differently depending on whether they pose an “unacceptable risk,” a “high risk,” or a “limited risk.” Applications that pose a clear threat to safety, fundamental rights, or democratic values fall into the “unacceptable risk” category and are strictly prohibited under the AI Act. Examples are social scoring by governments, real-time biometric identification in public spaces, and predictive policing systems. Applications that have a significant impact on people’s safety, rights, or livelihoods fall into the “high-risk” category. These systems are not forbidden but are subject to stringent transparency requirements and need to go through conformity assessments to ensure their responsible use.
Examples are AI systems that manage critical infrastructure, determine access to education, or are used in recruitment processes. AI applications that are thought to not have the same impact on safety or fundamental rights as high-risk systems, e.g., chatbots, recommender systems, and video editing tools, fall into the “limited risk” category. These applications require compliance with basic transparency obligations to ensure their ethical and transparent use. The AI Act empowers regulatory bodies, led by the newly created EU AI Office, to monitor compliance with the Act and enforce regulations, with steep fines for violations.
In contrast to the EU, the United States currently does not have comprehensive, federal AI-specific regulation akin to the EU AI Act. Instead, AI applications are primarily regulated through existing laws and sector-specific regulations in areas such as healthcare, finance, transportation, and employment. Additionally, there are state-level laws, e.g., the California Consumer Privacy Act and the Illinois Biometric Information Privacy Act, that indirectly regulate AI systems and applications. At the federal level, the National AI Initiative Act of 2020 aims to advance AI research, development, and use in a coordinated and strategic manner across the country to ensure that the United States remains a global leader in AI. Moreover, federal agencies such as the National Institute of Standards and Technology (NIST) and the Federal Trade Commission have released guidelines for assessing and mitigating AI risks. There are also multiple voluntary ethical guidelines adopted by major tech companies such as Microsoft, Google, and OpenAI. (For a more detailed analysis of the evolving US regulatory landscape and its sector-specific approach, see the following chapter which provides insights on recent policy shifts and their implications for AI developers.)
In Asia Pacific, China and Singapore stand out as the two countries that have been most active in regulating AI. Policy and governance initiatives in China are led by the central government and are aimed at maintaining rapid technological development while ensuring state control and alignment with the Chinese Communist Party’s political and social priorities. Key pillars of China’s AI governance framework include the New Generation Artificial Intelligence Development Plan, the Internet Information Service Algorithmic Regulation Provisions, the Data Security Law and Personal Information Protection Law, and the Ethical Guidelines for AI. China has also enacted targeted regulations for specific high-impact AI applications such as facial recognition, deepfakes, and autonomous vehicles. Overall, China’s AI governance landscape reflects the country’s ambition to lead globally in AI development while maintaining strict control over AI’s social and political implications.
Singapore’s approach to AI governance is guided by the Model AI Governance Framework, first introduced in 2019 and updated in 2020 by the Infocomm Media Development Authority and the Personal Data Protection Commission. Key principles meant to be advanced through the Model Framework are human-centricity, transparency, fairness, and accountability. The framework provides practical guidelines for businesses to deploy AI responsibly, focused on ensuring explainability, robustness, and stakeholder involvement. Singapore encourages organizations to conduct self-assessments using the Model Framework and the AI Verify toolkit, introduced in 2022. Overall, Singapore aims to balance regulation with growth and to be a global leader in trusted AI adoption.
Other notable AI policy and governance initiatives include Canada’s AI and Data Act (AIDA) and Brazil’s draft AI bill. Canada’s AIDA, introduced in 2022, aims to ensure AI systems are designed and used responsibly, with a focus on transparency, fairness, and accountability. It proposes oversight mechanisms for “high-impact AI systems” and establishes penalties for non-compliance, emphasizing trust in AI while promoting innovation. Brazil’s draft AI bill similarly seeks to establish a comprehensive framework for regulating AI, emphasizing ethical principles such as transparency, accountability, and human rights protection. The proposed legislation is aligned with international practices and aims to foster AI development while safeguarding public trust and preventing harm.
While these regulatory frameworks represent significant steps toward governing AI, each approach comes with its own set of strengths and limitations that merit closer examination.
3.2.2 Strengths and Weaknesses of Current Regulatory Frameworks
Strengths
Comprehensive frameworks such as the EU AI Act provide legal certainty and clarity to companies designing, developing, or using AI. The EU might also play a global leadership role if a “Brussels Effect” materializes in which other countries adopt legislation that is similar to the AI Act or global companies find it too costly to adapt their AI systems to different regulations and therefore conform to the strictest regulation globally. Another advantage of the AI Act is its risk-based approach, which balances innovation with safety by putting limits on the use of risky systems while ensuring that low-risk systems can be developed and deployed freely.
Weaknesses
The various policy and governance approaches employed by different jurisdictions may lead to fragmentation in global regulations that create compliance challenges for multinational organizations. In the worst case, the world will split into two (or more) technological blocs, which not only raises transaction costs for multinational companies but also increases the likelihood that the world will witness the emergence of AI systems that threaten human rights and fundamental freedoms.
There is also a risk that rapid technological advancements will outpace regulatory efforts. This is especially true for generative AI (GenAI), which has seen huge progress in the last 18 months alone. An additional challenge lies in the fact that many high-level AI governance principles (such as the ones contained in the EU AI Act) need to be operationalized through the development of technical standards, which not only is a slow and challenging process but also gives private actors significant control over how AI regulations are practically applied. This means that powerful interests represented in standard-setting organizations might have undue influence on the regulation of AI.
Given these strengths and weaknesses in regional approaches, the need for international coordination becomes increasingly apparent, though achieving global consensus presents its own set of challenges.
3.3 International Cooperation
There are many efforts at the regional and global levels to cooperate internationally on AI policy and governance. However, countries’ efforts to agree on truly “global” AI governance frameworks are hampered by geopolitical tensions.
3.2.1 The Need for Global Collaboration
Foundation models and small-scale AI as a service travel easily across borders, as they are digital products that can be accessed by anybody with a computer (and, if need be, a VPN connection). Thus, it is hard for governments to stop dangerous or otherwise unwanted AI from being used within their territories. This means that rather than trying to keep out risky AI, governments would be better off entering into global agreements that prevent dangerous AI from being developed or deployed in the first place. Such global agreements would also help to avoid fragmentation and would mitigate the risk of regulatory arbitrage, in which companies leave highly regulated markets to exploit less stringent jurisdictions.
3.3.2 Existing Collaborative Efforts
International collaboration on AI governance is critical to addressing global challenges and ensuring ethical, trustworthy AI systems. Among key joint efforts, the EU, United States, and United Kingdom are increasingly focused on fostering cooperation in regulating foundation models, such as large language models (LLMs). This includes initiatives to harmonize standards, share best practices, and align regulatory approaches, particularly on competition issues like market concentration and ensuring open and fair access to AI technologies.
The OECD Principles on AI, adopted by over 40 countries, provide a global baseline for trustworthy AI governance. These principles emphasize human-centric development, fairness, transparency, and accountability while fostering innovation. They serve as a foundation for international collaboration, enabling governments to implement consistent policies that support ethical AI.
UNESCO’s Recommendations on Ethical AI Development add a complementary dimension, focusing on the societal and cultural implications of AI. These recommendations advocate for inclusion, non-discrimination, and sustainability, with a strong emphasis on protecting human rights and promoting education to ensure equitable AI adoption globally. Together, these frameworks reflect growing global recognition of the need for coordinated governance to maximize AI's benefits while addressing risks effectively.
Despite these collaborative efforts, fundamental differences in national priorities and regulatory philosophies create significant obstacles to developing truly global governance frameworks for AI.
3.3.3 Challenges to Cooperation
Despite growing collaborative efforts, significant challenges persist due to diverging priorities and regulatory approaches across regions. The EU emphasizes a human-centric framework, prioritizing human rights, transparency, and accountability, exemplified by its AI Act, which imposes stringent requirements on high-risk AI applications. This strict regulatory environment compels companies operating in or entering the European market to adopt rigorous compliance measures, often necessitating significant adjustments to their AI models and operational practices to ensure transparency and accountability.
In contrast, China’s approach, which focuses on leveraging AI for economic growth and state control, aligns with its “core socialist values” and prioritizes national security over individual privacy. This regulatory stance necessitates that companies operating in China align their AI strategies with government policies and objectives, which may involve compromises on data handling and user privacy.
Meanwhile, the United States adopts a light-touch, innovation-first approach, favoring voluntary guidelines and sector-specific regulations to maintain its competitive edge in AI development. This environment allows companies greater flexibility in innovation and faster deployment of AI technologies but requires them to navigate a mosaic of state-level regulations alongside federal guidelines, which can vary significantly and impact scalability and uniformity in AI applications.
These differences often lead to disagreements on critical issues such as data protection, algorithmic transparency, and the role of state oversight. For instance, while the EU advocates for strict safeguards to prevent AI misuse, other regions may prioritize economic growth or geopolitical interests, creating friction in setting global norms. This fragmented regulatory landscape compels companies to develop adaptable and region-specific strategies for AI deployment, often requiring a localized approach to compliance and product development to meet diverse regulatory expectations.
Moreover, the lack of a unified enforcement mechanism further complicates collaboration, as countries pursue domestic policies that may conflict with international frameworks. This situation highlights the need for greater alignment on shared values and objectives to effectively address the global challenges posed by AI. For the moment, joint efforts at setting technical AI standards prove much more fruitful than attempts to create global ethical AI governance frameworks, suggesting a strategic focus for companies on focusing on technical standard settings that might offer some consistency across different markets.
Beyond the geopolitical and regulatory divergences, the development of ethical frameworks represents another crucial dimension of AI governance that addresses the technology's profound societal implications.
3.4 Ethical Considerations
AI has the potential to bring great advancements to humanity, but it also poses difficult ethical challenges. For one, AI itself can be biased and intransparent, and the data needed to train it can violate privacy rights or copyrights. AI can also be used for malicious ends (e.g., for the mass surveillance of populations by authoritarian governments). Increased recognition of these ethical pitfalls has led to numerous efforts to craft tools for ethical AI governance for, and by, public and private organizations.
3.4.1 Key Ethical Principles
The rise of AI has brought several urgent ethical issues to the forefront. These include:
Transparency: AI is increasingly being leveraged for important decisions by public and private organizations, including in sensitive contexts such as law enforcement, financial lending, and hiring. This makes it highly important that such decisions are transparent and can be explained to those affected.
Accountability: When AI systems cause harm, someone must be held liable. Establishing such liability is no trivial matter, however, as it could be argued that responsibility for the harm rests with either the developer or the user of the AI system or perhaps even with the AI system itself.
Fairness: Researchers have shown that AI can exhibit bias (e.g., against minorities) and therefore lead to discriminatory outcomes. This lack of fairness stems from existing biases in the training data, which are perpetuated by AI. However, several initiatives are underway to prevent, recognize, and mitigate the prevalence of bias in AI systems, thereby increasing the fairness of such systems.
Privacy: As mentioned above, AI raises issues around user privacy because the data used to train AI systems might include personally identifiable information. Safeguards ensuring that such information is not used or abused are an important component of fostering ethical AI.
3.4.2 Understanding Ethical Risks
Bias in AI models can lead to discriminatory outcomes. This is true for both predictive and GenAI. In predictive AI used in law enforcement contexts, for example, biases can lead to certain ethnic groups being stigmatized and, therefore, receiving harsher punishments. In GenAI used to answer questions, for example, responses can reflect existing negative stereotypes about women or minorities. Initiatives are underway to minimize such biases and their impact. For predictive AI, this means putting safeguards and limits on how such AI is used (especially in sensitive settings such as law enforcement and hiring). For GenAI, initiatives mostly focus on removing biased data from training sets.
Another danger related to GenAI is the proliferation of misinformation amplified by unregulated generative content. For example, AI can be used to churn out huge amounts of political propaganda that is then spread on social media platforms such as Instagram or TikTok. Efforts to address this danger mostly focus on social media platforms at the moment (which, in many cases, are also the developers of powerful AI systems). Thus, the EU in 2020 introduced the Digital Services Act, which imposes additional transparency and content moderation requirements on large digital platform companies.
3.4.3 Tools for Ethical Governance
Standard-setting organizations around the world have been busy drafting standards for the ethical governance of AI. Thus, the International Standardization Organization (ISO) and the International Electrotechnical Commission (IEC) developed a joint AI management standard in 2023 (ISO/IEC 42001) that “specifies requirements for establishing, implementing, maintaining, and continually improving” an AI Management System within organizations. The standard has been lauded for fostering responsible and ethical AI management but has also been criticized by some for being too complex for small organizations to implement.
Another tool for ethical governance is the American NIST’s voluntary AI Risk Management Framework. The framework emphasizes the promotion of trustworthy and responsible development and use of AI technologies. While the framework supports organizations in aligning their AI systems with ethical standards and regulatory requirements, it remains a voluntary standard without penalties for non-compliance.
While these standards and frameworks provide valuable guidance, their effective implementation ultimately depends on how organizations incorporate them into their governance structures and day-to-day operations.
3.4.4 Role of Organizations
Private organizations play a crucial role in the ethical development and deployment of AI, necessitating a multifaceted approach to governance and oversight. Internally, companies can establish ethical boards dedicated to continuous monitoring and evaluation of AI practices. These boards are instrumental in identifying and mitigating harmful practices before they lead to widespread consequences. Beyond internal governance, the adoption of technical controls such as automated red-teaming is critical. This process involves simulating cyberattacks to proactively identify and rectify vulnerabilities, ensuring systems are fortified against potential exploits.
Moreover, the responsibility of private organizations extends to rigorous compliance with regulatory standards. By aligning their operations with national and international legal frameworks, companies not only adhere to legal mandates but also contribute to the trustworthiness and reliability of AI technologies. Collaborative engagements with regulatory and governing bodies are also vital. Through partnerships, information sharing, and joint initiatives, private entities can influence policy-making processes, promoting regulations that reflect practical industry insights and ethical considerations.
By implementing these practices, organizations not only enhance their defensive capabilities but also position themselves as leaders in promoting ethical AI. This proactive stance in governance, compliance, and collaboration with regulatory authorities underscores their pivotal role in shaping an AI landscape that is secure, ethical, and beneficial for all stakeholders.
As ethical frameworks continue to evolve alongside the technology, forward-looking policy approaches must balance regulatory certainty with the flexibility needed to address AI's rapid advancement.
3.5 Future Policy Directions
Since AI is a rapidly changing technology, government decision-makers need to be willing to change their policies on a regular basis as well in order to keep pace with technological developments.
3.5.1 Adaptive and Dynamic Regulations
Given the fast pace of development of AI, there is a real need to design future-proof legislation that evolves with technological advancements. The EU has recognized this and is taking a flexible approach under the AI Act that allows for updates of the Act in response to technological changes without the need for a complete legislative overhaul.
AI can also be used to monitor compliance and enforce governance standards; there are, therefore, great hopes for Regulatory Technology (RegTech), which helps businesses comply with regulatory requirements more efficiently, increase regulatory agility, which in turn creates resilient societies that can handle the changes, and “shocks” stemming from the introduction of new technologies.
3.5.2 Focus Areas for Future Policies
There are several important focus areas for future policies to ensure that AI’s benefits can be harnessed while its risks are minimized.
With the rapid rise of GenAI, it has become pressing to draft regulations that address the unique risks of GenAI. For example, GenAI systems, like those used for creating images, videos, or text, can produce content that is indistinguishable from content produced by humans. This raises unique risks related to authenticity, misinformation, intellectual property rights, and more. Content watermarking is one of the proposed solutions to mitigate such risks. It involves embedding a digital marker or a signature into the content generated by AI systems. This fosters transparency and traceability, intellectual property protection, and regulatory compliance.
It is also crucial to strengthen cybersecurity requirements for high-risk applications given the sensitive nature of such applications. The consequences of attacks on such systems could be dire; think, for example, of hackers attacking an AI system used to determine access to educational opportunities.
Finally, it is important to expand liability frameworks to cover emerging use cases such as autonomous systems. Examples of such systems include self-driving cars and autonomous drones. Determining who is responsible when an autonomous system causes damage or harm can be complex. Is it the manufacturer, the software developer, the user, or some combination of these?
While these focus areas address critical risks associated with AI development, they must be balanced with measures that preserve innovation and technological progress.
3.5.3 Encouraging Innovation While Ensuring Safety
Regulations are important to manage the risks of AI. However, regulations also need to be balanced with incentives for innovation:
Regulatory sandboxes can be used to test new technologies in controlled environments. These sandboxes provide a structured context where AI technologies can be deployed to assess their impact, effectiveness, and potential risks without the full burden of regulatory compliance. This helps innovators and regulators understand the technology in a practical setting.
Funding programs like the EU’s Recovery and Resilience Facility can help support trustworthy AI development. This program supports economic recovery in European countries and aims to bolster investment in technologies that are ethically sound and reliable.
Arm navigates global regulations by combining adherence to compliance requirements with active participation in shaping regulatory frameworks. This strategic approach involves adapting to diverse regulatory environments across different jurisdictions and engaging in discussions that influence the formulation of new laws. A notable initiative in this context is the advocacy for regulatory sandboxes, which allow for the testing of new technologies under controlled regulatory conditions, minimizing exposure to the broader market's regulatory complexities. Such measures facilitate Arm's management of regulatory risks and contribute to its role in the discourse on AI policy and practice, reflecting a blend of compliance and strategic engagement.
3.6 Conclusion
AI has the potential to be highly beneficial for humanity, but there are also serious risks attached to the rise of AI. Policy and governance are, therefore, critical for shaping a safe, ethical, and innovative AI ecosystem. The ethical principles discussed—transparency, accountability, fairness, and privacy—provide essential frameworks for responsible AI development. Particularly large benefits are to be gained through international cooperation on AI governance that results in harmonized standards benefitting society globally. Such harmonized standards not only lower transaction costs for globally operating companies but can also help foster AI that advances human rights and fundamental freedoms. Arm has an important role in fostering compliance-ready technologies that align with evolving regulatory requirements. The next chapter provides a practical perspective on how these regulatory frameworks affect businesses and developers on the ground, including valuable insights into navigating today's complex AI governance landscape while maintaining innovation.
Arm Sidebar: AI Policy, Regulation, and Global Trends
By Vince Jesaitis, Senior Director, Government Affairs, Arm
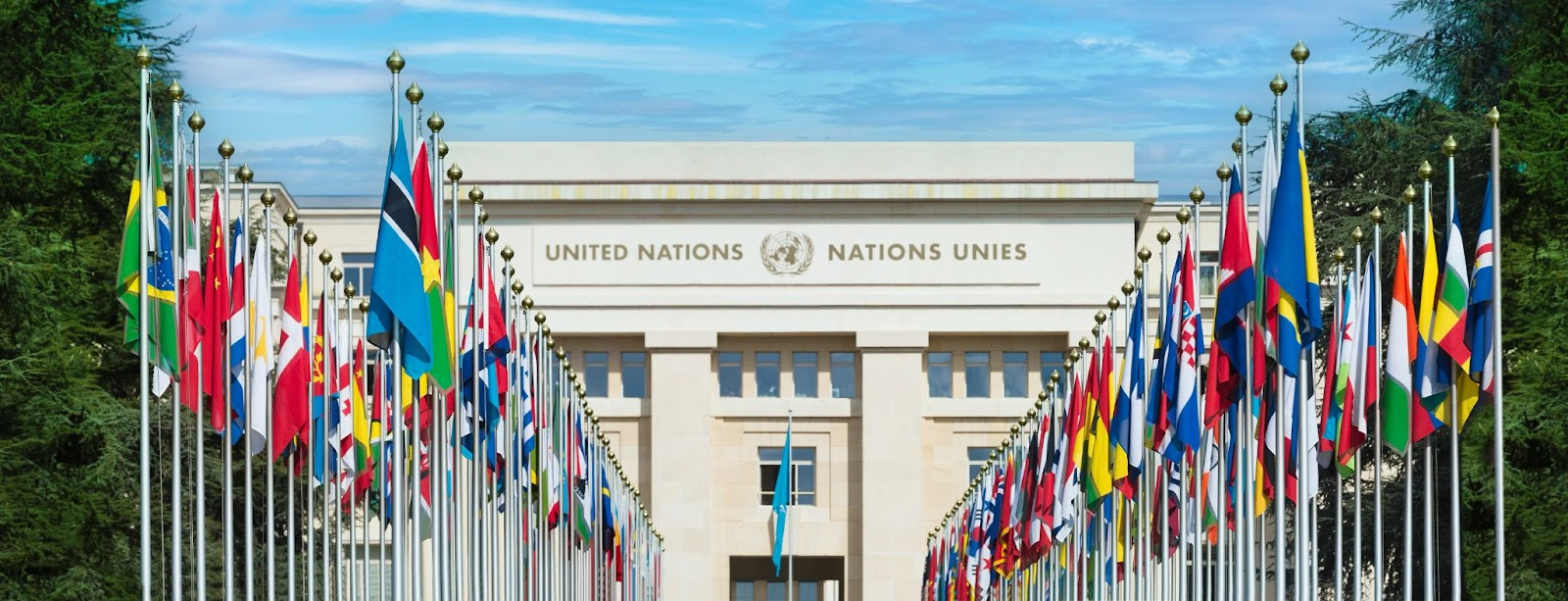
I use AI daily to search and summarize emails, create first drafts of emails, and work on otherprojects, and yes, even to summarize AI policy and regulations being released by local and national governments. Yet, it appears businesses are taking a cautious approach. They worry about risks like misuse or unintended consequences. Historically, governments have intervened in times like these to establish "rules of the road" for industries such as automotive, pharmaceuticals, and chemical production to address safety concerns. However, a lack of technical understanding and consensus on potential harms has limited government action and harmonization in these areas. The absence of consistent regulatory frameworks only adds to business hesitation, as global efforts struggle to keep up with AI’s rapid evolution, or even commonly define the risks.
While the vast majority of AI use cases are low risk, some present significant challenges. The latter is where most governments should and generally are focusing. Recent reports and discussion highlight how AI systems often function as a “black box,” making it difficult to understand the reasoning behind their decisions or predictions. This lack of transparency can lead to unintended consequences, like unfair hiring practices or denying public benefits, with no clear way to pinpoint or fix the underlying issue.
Beginning to recognize these risks, many governments around the world are pushing for AI regulation aimed at preventing disruptive impacts—whether to businesses, data privacy, public programs, or national security. These efforts underscore the need for every industry to stay informed about AI advancements and regulation to ensure they are maximizing the benefits of the technology, while avoiding potential downsides or harm.
However, not everyone I know agrees on the urgency of regulation. Many AI developers argue that the risks are overstated and that premature or overly restrictive rules could stifle innovation. They worry that complex, uninformed, vague, or misaligned regulations may hinder progress without effectively addressing the risks they aim to address, especially given the rapid pace of AI development.
Proponents of regulation argue that the unchecked growth of AI could lead to significant harm, and in some cases, it already has. For instance, generative AI tools are being used to create convincing fake images and videos, fueling the spread of misinformation online and non-consensual likeness content. Without proper oversight, these risks could undermine trust, disrupt industries, and severely harm individuals on a large scale.
As the debate continues, one thing is clear: businesses, governments, and developers alike must find a balanced approach to addressing AI regulation. This means gaining a deep understanding of the technology and fostering innovation while addressing the risks, ensuring AI serves as a tool for progress rather than a source of harm.
The Global Divide on AI Risks
Globally, there’s little agreement on the risks AI poses, which makes establishing unified regulations incredibly challenging. Each country approaches AI safety through its own unique lens, shaped by its priorities and perspectives. However, there is more agreement among nations with established AI safety institutes, such as the U.K., Japan, and South Korea, where alignment on AI risks is stronger.
Then, there’s the Organization for Economic Cooperation and Development (OECD, which includes a broader group of higher-income, predominantly Western countries. While there is still less agreement than in the first group, these countries share more common ground on AI regulation. The least agreement, however, can be found within the UN General Assembly, where countries like Saudi Arabia, China, Malaysia, Iran, Rwanda, the U.S., and Brazil sit side by side but have vastly different views on the risks AI poses.
What to Expect From Shifting AI Regulation in the U.S.
AI regulation in the U.S. is undergoing significant changes. The Trump administration is avoiding broad AI regulations, contrasting sharply with Europe’s sweeping initiatives like the EU AI Act (as explored in more detail in the previous section). This position was evident in his first several days in office in which he deregulated many industries, including the technology sector.
The Trump administration also rescinded the Biden administration’s AI executive order, including plans for the U.S. AI Safety Institute, which aimed to reduce risks to consumers, workers and national security. Instead of a comprehensive federal framework for AI governance, the focus shifted to minimal intervention, creating a more open environment for AI development.
Sector-Specific vs. Cross-Sectoral AI Regulation
In the U.S., we've historically taken a sector-specific approach to regulation, and I expect AI to follow this trajectory. In fact, incoming Senate Commerce Committee Chair Ted Cruz recently emphasized that policymakers should avoid getting in the way of innovation and that AI legislation should address specific problems with narrowly focused solutions. Unlike the EU AI Act, which imposes broad, cross-sectoral restrictions that can delay or block AI services regardless of their purpose, U.S. regulations are likely to address AI use in clearly defined contexts. For example:
Personal harms: Banning dissemination of images or videos with non-consensual name, image, and likeness (NIL).
Finance: Defining guardrails for AI in banking, stock trading, and other financial services.
Healthcare: Establishing guidelines for AI used in diagnostics, such as detecting cancer in medical imaging.
This tailored approach allows for flexibility within industries but stops short of overarching restrictions.
The Role of States in AI Regulation
With no comprehensive federal AI regulations, states are stepping in to fill the gap. California’s Senate Bill 1047 signals a growing trend of state-level action, similar to how privacy laws evolved in the U.S., with federal laws targeting specific sectors, and states like California introducing broader protections. Colorado has also enacted the first AI law focused on high-risk systems, effective February 1, 2026. Both the CAIA and EU AI Act adopt a risk-based approach and emphasize transparency and data governance, though the EU AI Act applies more broadly and includes obligations not covered by the CAIA.
While the U.S. federal government focuses on targeted oversight, I see the resulting patchwork of state-level regulations adding complexities for businesses operating across jurisdictions. In California alone, by the end of the fiscal year in September, 38 separate AI bills will await the Governor’s approval, including one addressing deepfakes in pornography. Companies will need to monitor both state and federal developments to ensure compliance. As the landscape evolves, the U.S. will likely continue to differ sharply from Europe in its approach, favoring innovation and flexibility over broad, comprehensive measures.
Challenges of Operating Under the EU AI Act
Harmonizing AI regulations across regions is a challenging task, largely due to differing mindsets. In Europe, AI is often seen as posing existential risks that must be addressed through strict oversight. The EU AI Act reflects this perspective, but its impact on non-European companies remains uncertain.
Unlike the General Data Protection Regulation (GDPR), which established significant extraterritorial influence due to the global flow of data, the EU AI Act has yet to reach the same level of international integration. While the Act includes extraterritorial provisions similar to GDPR, its scope and enforcement remain less clear, leading some companies to avoid compliance altogether. For example, Meta recently decided not to roll out certain AI tools in the EU, citing uncertainty about meeting regulatory requirements. This mirrors early GDPR responses, where major companies like Google focused on assessing fines and compliance workarounds rather than aligning fully with the law. Regulating AI is far more complex because it focuses on overseeing transformative technology rather than specific behaviors, marking a significant shift in global regulatory approaches.
However, the stringent requirements of EU regulations have sparked concerns about their practicality and long-term impact. For instance, the recently passed Cyber Resilience Act imposes highly specific—and arguably excessive—standards on technology providers. One notable example is a rule requiring mobile phones to withstand being dropped from three feet 48 times to qualify for sale in the EU. For folding phones, the number drops to 35. These extreme and overly specific requirements risk pushing consumers to source products outside the EU.
A Shift in AI Regulatory Mindset
Recognizing these challenges, there are signs that EU leadership is reconsidering its regulatory approach. The new European Commission leadership has stated it wants to reevaluate the regulatory actions of past governments. The President of the European Commission, Ursula von der Leyen, has suggested pausing further regulation to evaluate whether existing policies have had the expected impact, or overly restricted the competitiveness of domestic industries without realizing the expected benefits. This reflection extends to the AI Act, as well as other regulations introduced over the past two decades, which some argue have limited the ability of European companies to compete on a global scale.
Implications for U.S. and Global Companies
For now, it seems unlikely that the EU AI Act will significantly hinder American, UK, or Chinese companies’ ability to operate in Europe to the same extent as GDPR. However, as the EU revisits and potentially revises its regulatory frameworks, U.S. companies will need to stay agile, balancing compliance with their global operational goals. This evolving landscape may ultimately offer opportunities for collaboration and innovation, particularly if the EU reevaluates its actions to balance regulatory action with competitiveness.
How Developers May Navigate AI Regulatory Frameworks
In an era of inconsistent AI regulation, developers must have a clear understanding of their products before bringing them to market. This means anticipating the long-term implications, potential risks, and possible harms associated with their innovations. While regulators may not prescribe exactly how to develop or market AI products, they are likely to establish mechanisms to ensure accountability. The goal is to prevent scenarios where a product may generate impressive results, such as creating a compelling image, but could also be misused for malicious purposes.
One approach I see in the EU and UK involves the use of regulatory sandboxes. These controlled environments allow developers to test their technologies under a protective framework, enabling experimentation without immediate exposure to liability or enforcement actions. However, once a product leaves the sandbox, it must comply with broader regulatory requirements.
In contrast, the U.S. is unlikely to adopt a broad sandbox-driven model, as US regulators are more focused on enforcement, which could lead to audits, actions, and public disclosures. Instead, a balanced approach could encourage innovation while addressing risks, allowing developers to bring products to market with ongoing monitoring and the flexibility to address issues as they arise, while ensuring safeguards against misuse.
Preparing the Workforce for the AI Revolution
Policymakers, government agencies, and the private sector have a critical role to play in addressing the economic and social implications of AI, such as job displacement and inequality. As industries transition to more AI-driven processes, it is essential to ensure that the population is equipped with the education, skills, and training needed to interact with emerging technologies.
This doesn’t mean training everyone to become computer scientists. For example, farmers in Idaho are beginning to use robots to monitor sections of their farms, checking soil temperature and moisture levels. While currently on a small scale, it highlights the importance of updating educational curricula to align with the evolving needs of various industries. Workers in fields ranging from agriculture to healthcare need foundational knowledge that enables them to effectively utilize these new tools to enhance their productivity.
As technology advances at an unprecedented rate, the need for lifelong learning beyond the traditional K-12 model is growing. In Finland, primary school children are already being taught how to spot AI deep fakes. Currently, much of continuing education is driven by the private sector, often leaving gaps for displaced workers. For instance, when automation disrupts industries and workers lose their jobs, they need accessible opportunities to gain new skills and re-enter the workforce, or ideally, to gain those skills continually before losing a job. Governments must create systems for ongoing education that extend beyond the current framework. By doing so, they can support workers through these transitions and help them adapt to the demands of an AI-driven economy.
AI Robots Will Change Regulatory Considerations
As we edge closer to the prospect of mass-producing robots, new AI regulations are emerging. Unlike software-based AI systems that operate in virtual spaces, robots bring AI into the physical world—interacting with people and environments in tangible, often unpredictable ways. In Japan, for example, many hotels already use robots to greet and check-in guests, helping to address the challenges of an aging population. This shift raises a host of complex regulatory questions.
When AI moves beyond digital interactions to power machines capable of physical actions, safety, accountability, and ethical use become critical concerns. Regulators must address liability for accidents, the security of autonomous systems, and the integration of robots into existing legal frameworks. New regulations, like the EU Product Liability Act, are shifting liability upstream in the supply chain to include not only manufacturers but also technology providers involved in creating the product.
The broad deployment of robots could reshape the regulatory landscape, compelling governments and industries to reconsider the boundaries and responsibilities of AI-enabled technologies in the physical world. If the timeline for this technological leap is as imminent as some suggest, including just recently at CES, industries must prepare for heightened scrutiny and a wave of policies designed to address the unique challenges posed by physical interactions with walking, talking, sensing and acting computers.
Balancing Sector-Based Regulation and Global AI Policy
A sector-based regulatory approach for AI makes sense because it allows for targeted regulations addressing specific harms in distinct environments. For instance, consumer-facing applications like ChatGPT involve unique risks that can be addressed within their domain. However, the same AI model used in a financial services context may present an entirely different set of challenges, requiring tailored regulations for that unique environment. By focusing on environment-specific risks, regulators can craft more effective and nuanced policies.
The challenge, however, lies in the lack of international agreement on this approach. Between jurisdictions, particularly the U.S. and Europe, there is often a fundamental distrust of motivations. The EU’s gatekeeper regulations, for example, target large companies with significant market share, most of which are U.S.-based, raising questions about fairness and intent. While Europe’s concerns about market power stifling competition have some validity, this tension underscores the difficulty of creating a unified global regulatory framework for AI.
Despite these challenges, there is cause for optimism. Governments worldwide are beginning to recognize the transformative potential of AI, taking more time to explore and understand the technology, and are investing in the necessary infrastructure to support it. Over the past year, there’s been a notable shift in focus from software and model development to the critical role of computing power. Policymakers in the U.S., Europe, Southeast Asia, and beyond are realizing that software capabilities depend entirely on hardware infrastructure. Without adequate compute capacity, the full benefits of AI cannot be realized.
This recognition has led to significant investments in compute infrastructure. Governments are dedicating substantial resources to ensure that their regions have the computational capacity to compete in the global AI race, and rapidly taking steps to ensure more compute power can be deployed in an expedited fashion. This shift mirrors the evolution of utilities like electricity, which became ubiquitous and essential. Similarly, AI is poised to become the next foundational layer of global infrastructure, akin to ubiquitous access to computing power via cloud platforms.
With the path to balanced regulation still unfolding, the acknowledgment by governments across the globe of AI’s potential—and the infrastructure needed to support it—signals a promising path forward. Just as governments didn’t immediately mandate seat belts, airbags, and backup cameras for the Model T, they need to stay informed about technological advancements and mitigate harm as technology develops. As policies and frameworks evolve, trial and error will play a role, but the trajectory suggests a future where governments and industries can harness AI's power responsibly and effectively.